These days I like to discuss
- Analytic Design Theory
- Statistical Communication
- The Casual Inference Podcast
- Large-scale medical data
- Italian
- Co-founding R-Ladies Nashville
- Disney World
over coffee
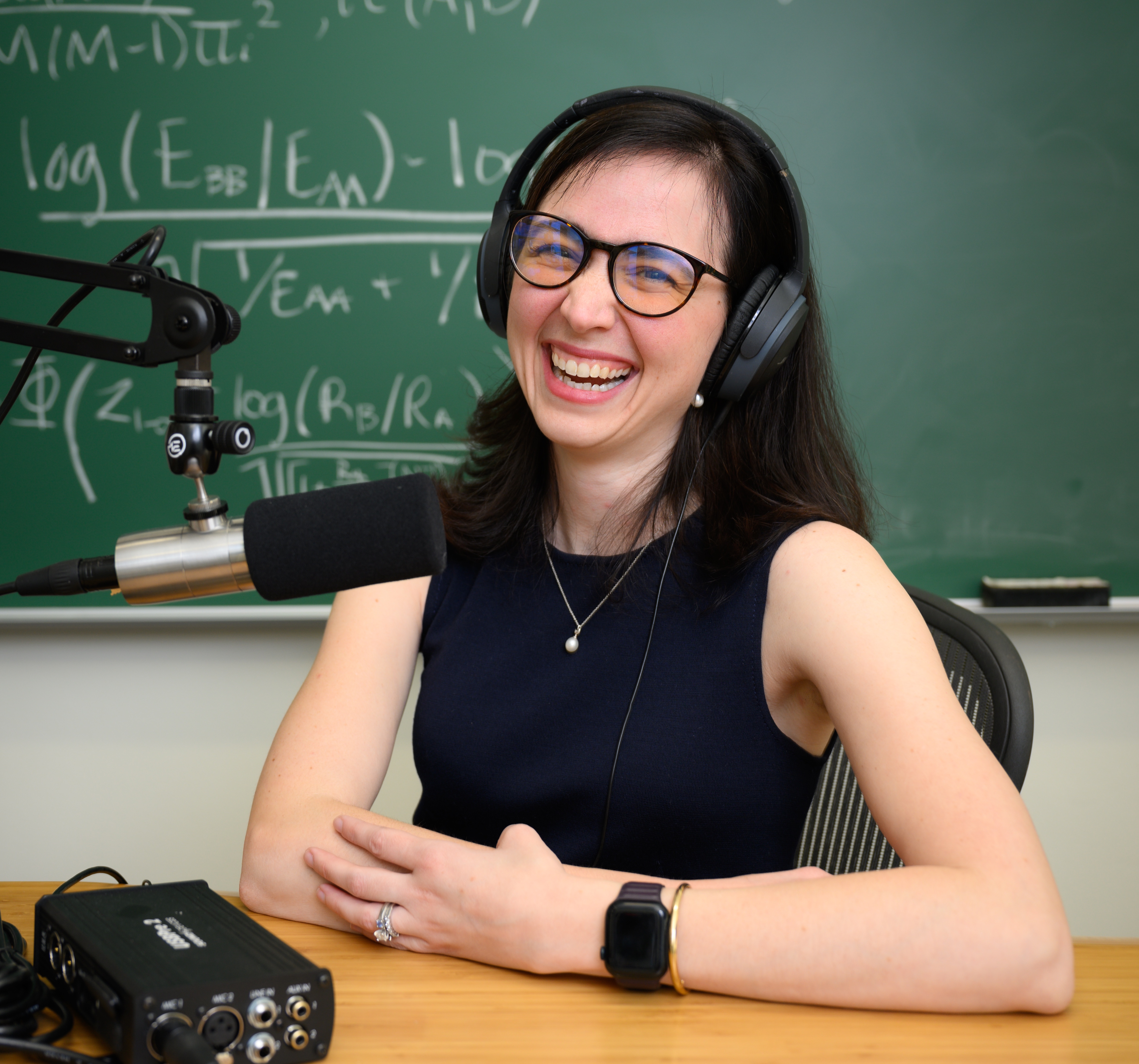
Lucy D’Agostino McGowan
Lucy D’Agostino McGowan is an assistant professor in the Department of Statistical Sciences at Wake Forest University. She received her PhD in Biostatistics from Vanderbilt University and completed her postdoctoral training at Johns Hopkins University Bloomberg School of Public Health. Her research focuses on analytic design theory, statistical communication, causal inference, and data science pedagogy. Dr. D’Agostino McGowan is the 2023 chair of the American Statistical Association’s Section on Statistical Graphics and can be found blogging at livefreeordichotomize.com, on Twitter @LucyStats, and podcasting on the American Journal of Epidemiology partner podcast, Casual Inference.
Awards
Lucy was selected for the Teaching in the Health Sciences Young Investigator Award for her paper Design Principles for Data Analysis. She was also selected as an ASA StatsForward Fellow.
Listen to the Casual Inference Podcast
Recent & Upcoming Talks
It's ME hi, I'm the collider it's ME
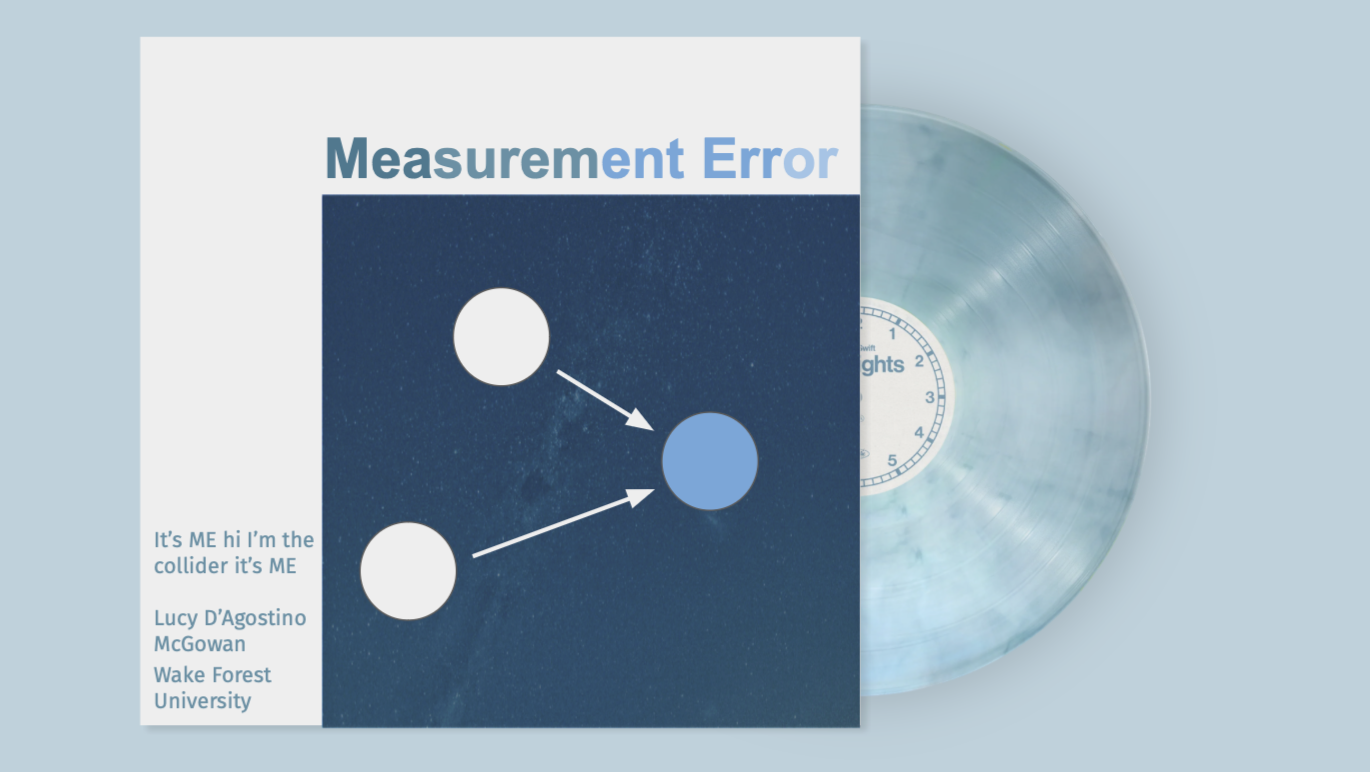
This talk will focus on framing measurement error as a collider from a causal inference perspective. We will begin by demonstrating how to visually display measurement error in directed acyclic graphs (DAGs). We will then show how these graphs can be used to help communicate when corrections for measurement error are needed and how to implement these corrections in order to estimate unbiased effects. Finally, we will demonstrate how sensitivity analyses traditionally used to address omitted variable bias can be used to quantify the potential impact of measurement error.
Read moreIncluding the outcome in your imputation model -- why isn't this 'double dipping'?
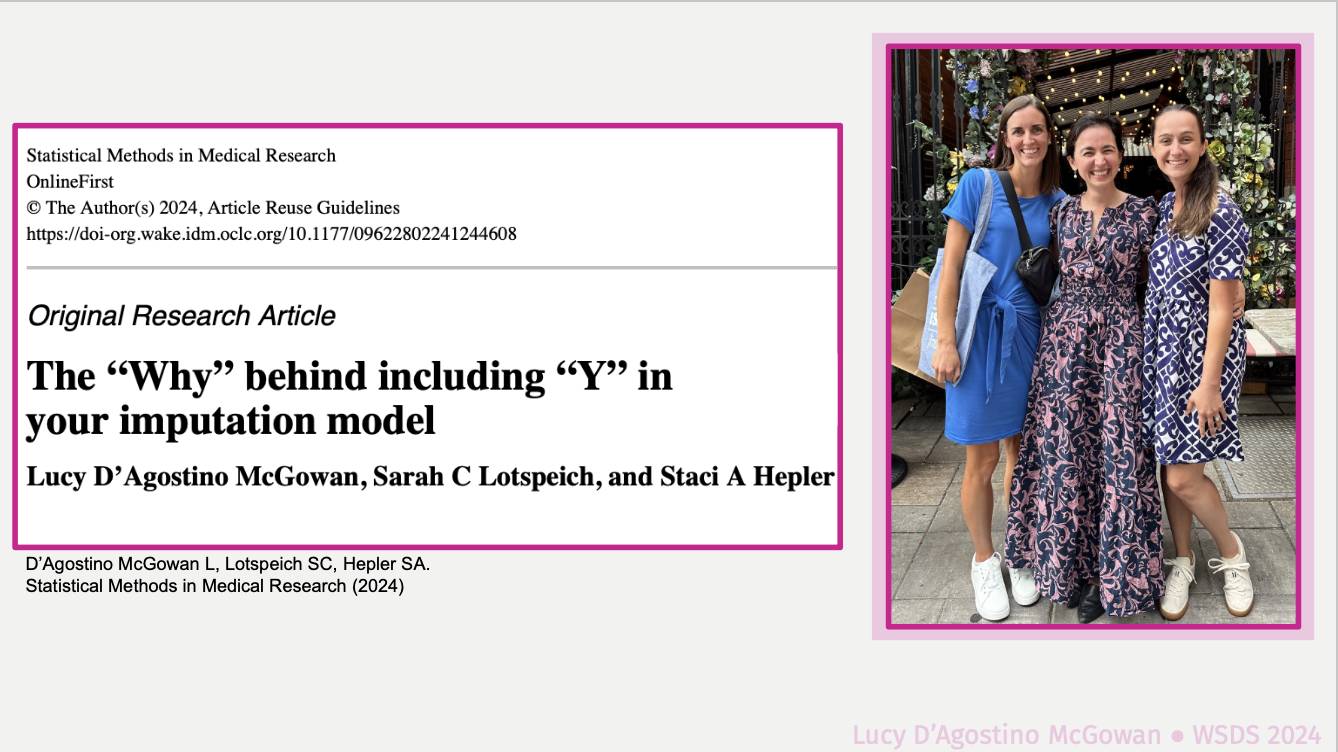
An often repeated question is whether including the outcome in an imputation model is ‘double dipping’ or ‘peeking’ at the outcome in a way that can negatively impact the Type 1 error in studies. This talk will dive into this myth and help dispel these concerns. We mathematically demonstrate that including the outcome variable in imputation models when using stochastic methods is required to avoid biased results. A discussion of these results along with practical advice will follow.
Read morePower and sample size calculations for testing the ratio of reproductive values in phylogenetic samples
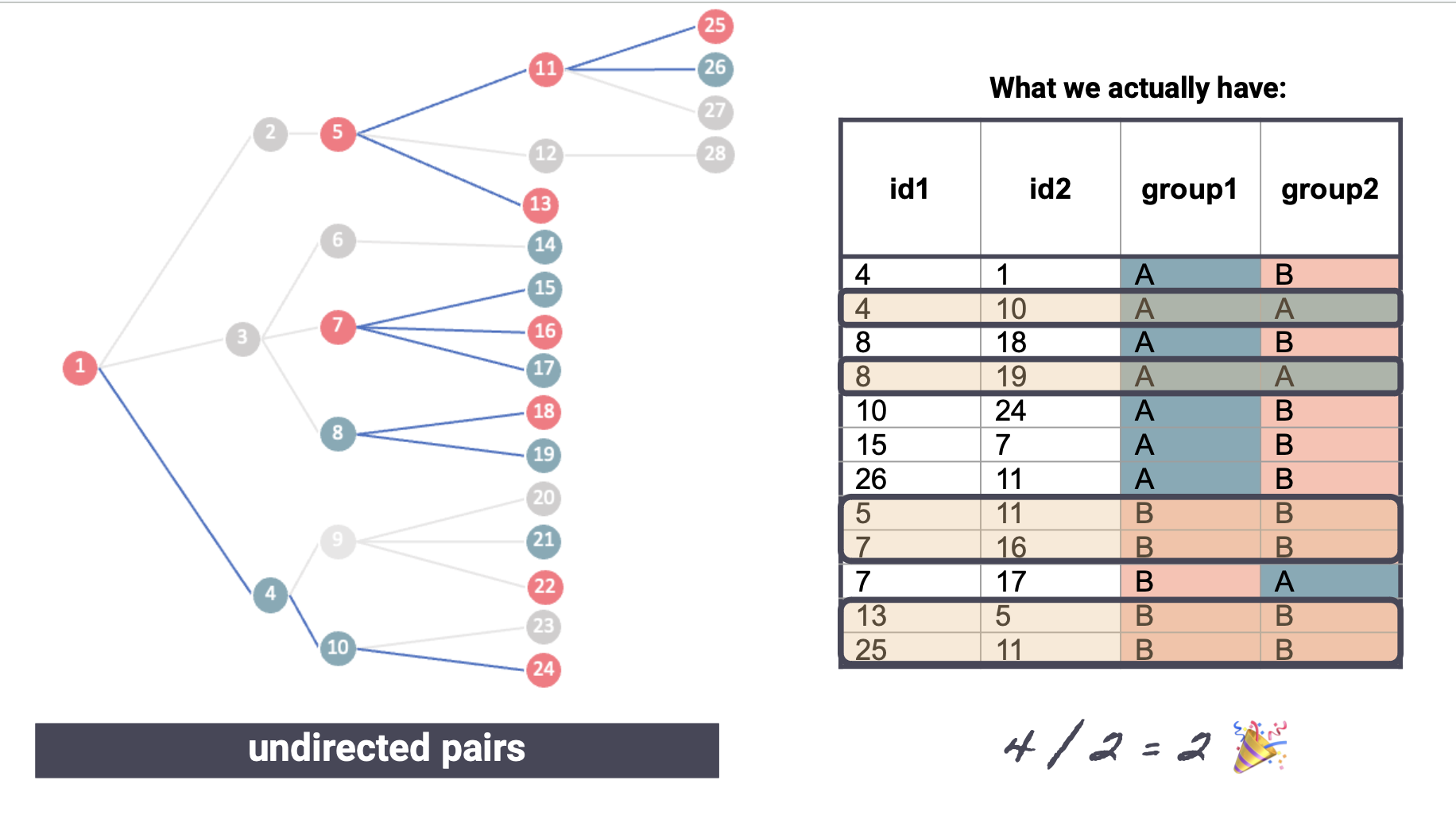
The quality of the inferences we make from pathogen sequence data is determined by the number and composition of pathogen sequences that make up the sample used to drive that inference. However, there remains limited guidance on how to best structure and power studies when the end goal is phylogenetic inference. One question that we can attempt to answer with molecular data is whether some people are more likely to transmit a pathogen than others. In this talk we will present an estimator to quantify differential transmission, as measured by the ratio of reproductive numbers between people with different characteristics, using transmission pairs linked by molecular data, along with a sample size calculation for this estimator. We will also provide extensions to our method to correct for imperfect identification of transmission linked pairs, overdispersion in the transmission process, and group imbalance. We validate this method via simulation and provide tools to implement it in an R package, phylosamp.
Read moreTeaching
STA 112 -- WFU Spring 2024
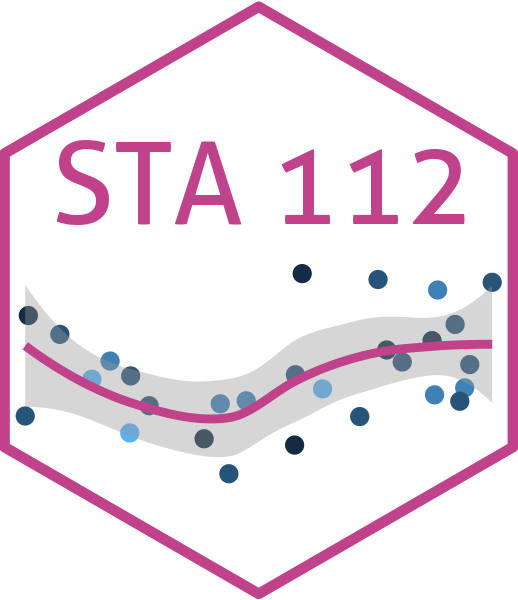
Introduction to Regression and Data Science. Learn to explore, visualize, model, evaluate, and communicate data in a reproducible manner. Gain hands on experience with real data from a variety of disciplines. The course will focus on the statistical computing language R.
Read moreBEM 392 -- WFU Spring 2024
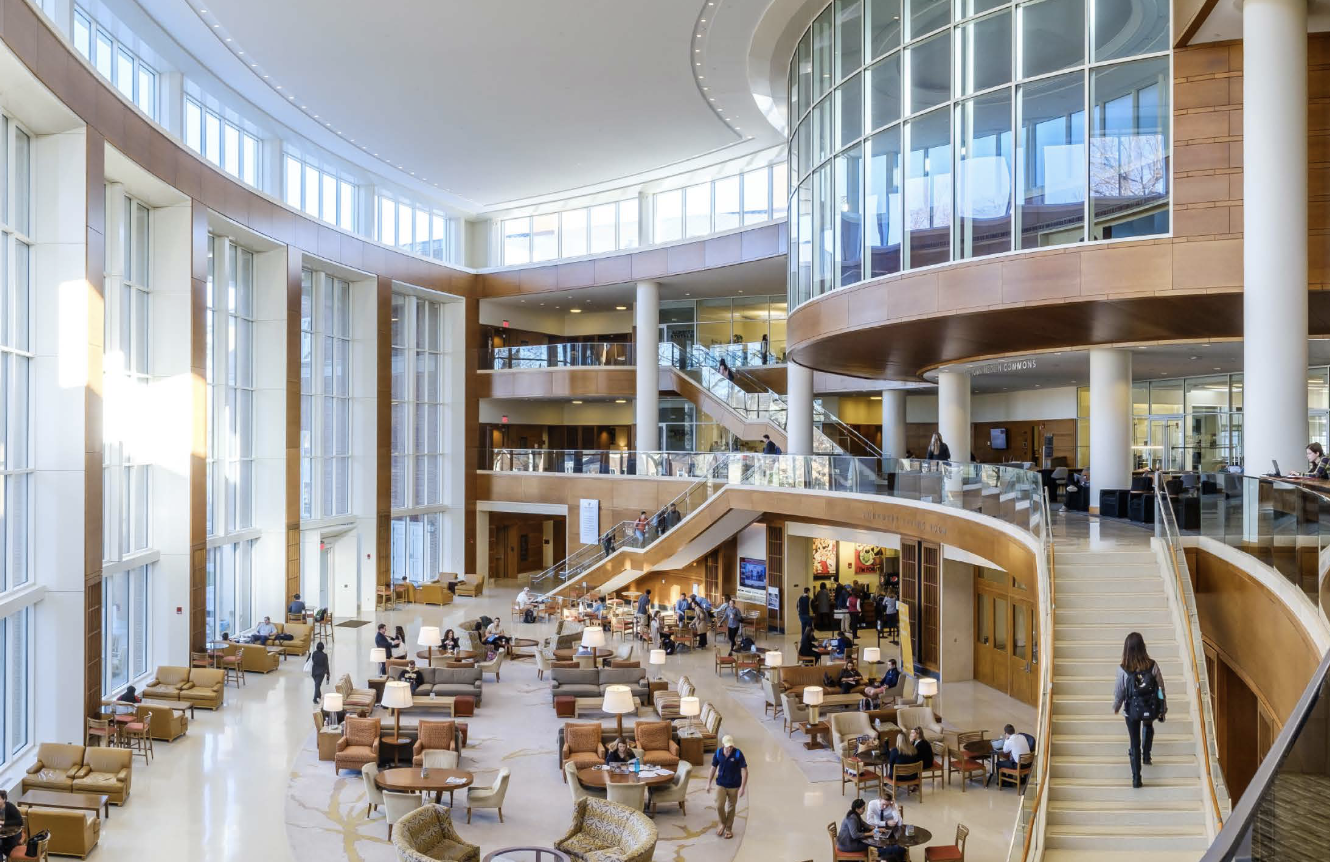
Seminar in Mathematical Business Analysis. The main purpose of this seminar is to develop the capability to apply quantitative knowledge to real and ill-defined problems. It tries to bridge the gap between the theory of quantitative decision approaches such as management science/operations research, information systems, and statistics (now mainly collected in the Business Analytics field), with the application of these approaches to the solution of actual business problems.
Read moreSTA 779 -- WFU Fall 2023
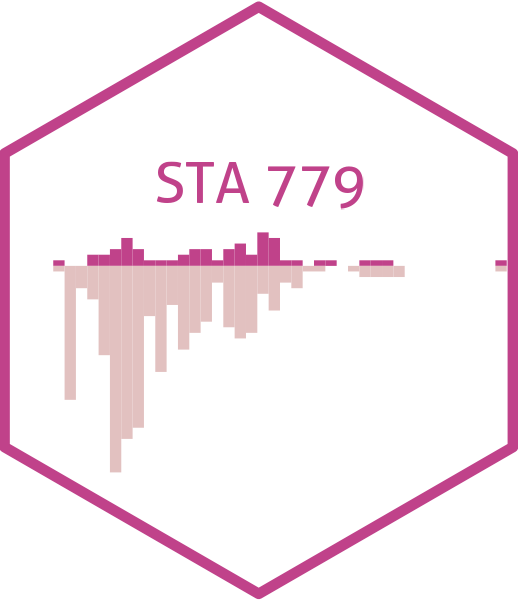
Causal Inference. From Correlation to Causation. The goal of this course is to give students the skills needed to conduct analyses and communicate results when causality is the goal. Students will learn how to implement causal inference techniques including matching and weighting, evaluate assumptions, and conduct sensitivity analyses.
Read moreWriting
Partnering with Authors to Enhance Reproducibility at JASA
The 'Why' behind including 'Y' in your imputation model
Missing data is a common challenge when analyzing epidemiological data, and imputation is often used to address this issue. Here, we investigate the scenario where a covariate used in an analysis has missingness and will be imputed. There are recommendations to include the outcome from the analysis model in the imputation model for missing covariates, but it is not necessarily clear if this recommendation always holds and why this is sometimes true.
Read morePower and sample size calculations for testing the ratio of reproductive values in phylogenetic samples
The quality of the inferences we make from pathogen sequence data is determined by the number and composition of pathogen sequences that make up the sample used to drive that inference. However, there remains limited guidance on how to best structure and power studies when the end goal is phylogenetic inference. One question that we can attempt to answer with molecular data is whether some people are more likely to transmit a pathogen than others.
Read more