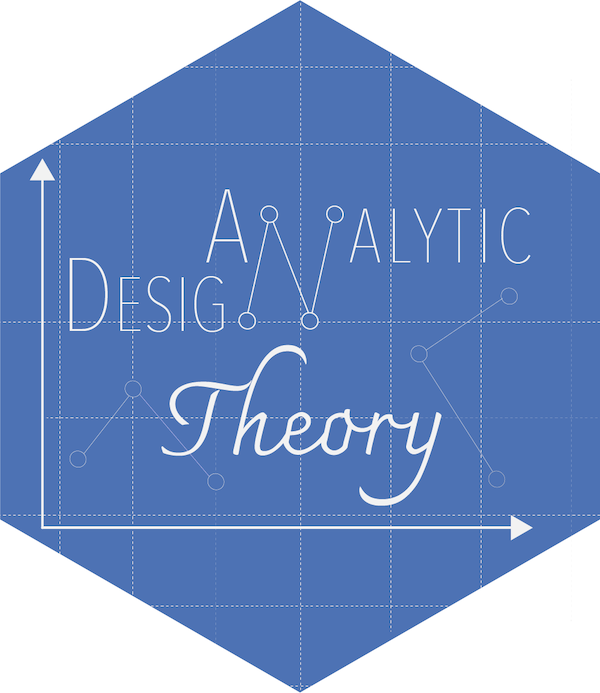
Design Principles of Data Analysis
The data revolution has sparked greater interest in data analysis practices. While much attention has been given to statistical thinking, another type of complementary thinking that appears in data analysis is design thinking – a problem-solving approach focused on understanding the intended users of a product. When facing a problem, differences arise in how data analysts construct data analyses, including choices in methods, tools, and workflows. These choices impact the analysis outputs and user experience. Therefore, a data analyst’s role can be seen as designing the analysis with specific principles. This webinar will introduce six design principles for data analysis and describe how they can be mapped to data analyses in a quantitative and informative manner. We also provide empirical evidence of variation of these principles within and between data analysts. This will hopefully provide guidance for future work in characterizing the data analytic process.